Deep learning works over complex hierarchical models to attain refined accuracy and optimized predictions. It utilizes the data inputs, weights, and biases to identify, classify, and describe the elements of the dataset. It is used in applications where analytical tasks and automation are needed without human involvement. This is why deep learning interview questions are mostly about neural networks and their applications in creating advanced models.
Deep learning models process the incoming unstructured data and perform automated feature extraction to enable self-learning. These advanced deep-learning neural networks have been successfully implemented in self-driving cars, digital assistants, news aggregation, and investment modeling.
To help you prepare for the next opportunity, we have listed all possible deep learning interview questions in one place. This article will help you understand what could be asked as Deep Learning and machine learning interview questions.
Top 50+ Deep Learning Interview Questions [With Answers]
[Updated]1. Why do we need Deep Learning when Machine Learning is present?
Machine learning is a framework that uses past data to identify relationships among features. Machines can then predict new data using mathematical relationships by generating dynamic, accurate, and stable models.
On the other hand, deep learning is a segment of machine learning that uses complex algorithms based on biological neural networks to mimic the human brain and make decisions and actions like humans.
The brain’s functioning and structure inspire Deep Learning to train neural networks. Deep learning also performs various complex operations to extract hidden features and patterns.
The critical aspect differentiating the two is that deep learning can extract hidden features and patterns in the data. In contrast, other machine learning can reduce dimensions but not identify new features.
Also read Machine Learning vs Deep learning- What Is the Difference?
2. What are the applications of Deep Learning?
The applications of Deep Learning bucketed according to the type of learning are:
The supervised tasks in deep learning are:
- Text Classification
- Sentiment Analysis
- Auto-Tagging System
- Image Classification
- Audio Classification
- Forecasting
Also read:
The unsupervised tasks in deep learning are:
- Image Segmentation
- Image Localization
- Image Captioning
- Object Detection or Identification
Also read: What is Image Segmentation?
The problems that involve generating data in deep learning are:
- Machine Translation
- Chatbots
- Speech to Text
- Recommendation Systems
- Automatic Text Generation
Also read: Top 15 Real World Applications of Artificial Intelligence
3. Name the Deep Learning frameworks and tools that you have used.
The deep learning frameworks and tools are:
- Keras
- TensorFlow
- PyTorch
- Theano
- CNTK
- Caffe2
- MXNet
4. List the commonly used data structures in deep learning.
The data structures used in deep learning are:
- Lists
- Dictionaries
- DataFrames
- Matrices
- Tensors
- Computation Graphs
5. What are the supervised and unsupervised learning algorithms in Deep Learning?
The supervised learning algorithms are:
- Artificial Neural Network (ANN)
- Perceptron (single and multi-layer)
- Convolution Neural Network (CNN)
- Recurrent Neural Network (RNN)
The unsupervised learning algorithms are:
- Autoencoders
- Self-Organizing Maps (SOMs)
- Boltzmann Machine
- Generative adversarial networks (GANs)
6. What are deep and shallow networks? Which one is better and why?
A shallow neural network has only one hidden layer, and a deep neural network has more.
Both networks can approximate any function. A shallow network requires more parameters and is limited by its layers, whereas a deep network can leverage its number of layers to compute efficiently and extract more abstract features.
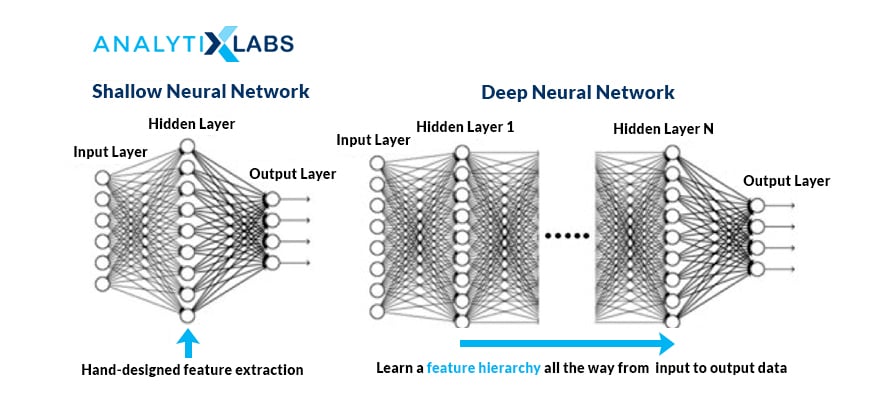
Source: cloudfront.net
7. What is the difference between Single-Layer and Multi-Layer Perceptron?
In a neural network, a perceptron is the processing unit that performs computations to extract features. It is the building block of the Artificial Neural Network (ANN).
A single-layer perceptron is the simplest neural network without any hidden layer. It is a linear classifier with only one neuron and can perform binary classification. It can only classify linear separable classes with binary output.
Also read: Understanding Perceptron – The Founding Element of Neural Networks
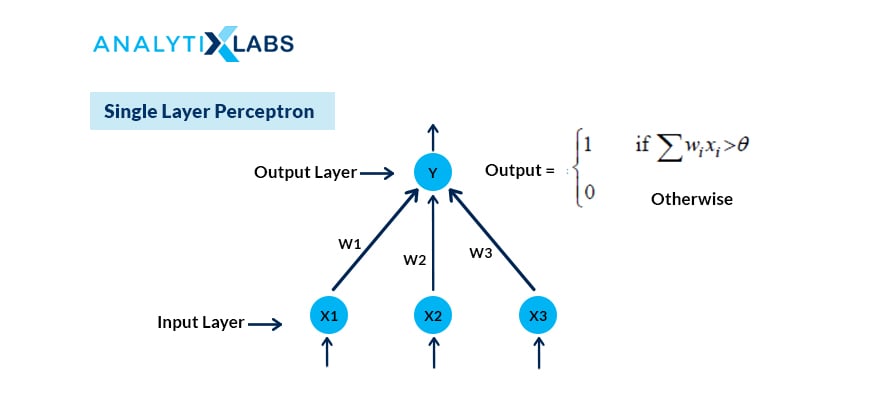
A multilayer perceptron (MLP) is another Artificial Neural Networks (ANN) class with more than one perceptron. MLP consists of three layers: input, hidden, and output. The input layer receives information, and the output layer makes the prediction or decision.
An MLP can have one or more hidden layers. It can classify the nonlinearly separable data points as hidden, and the output layer uses a nonlinear activation function.
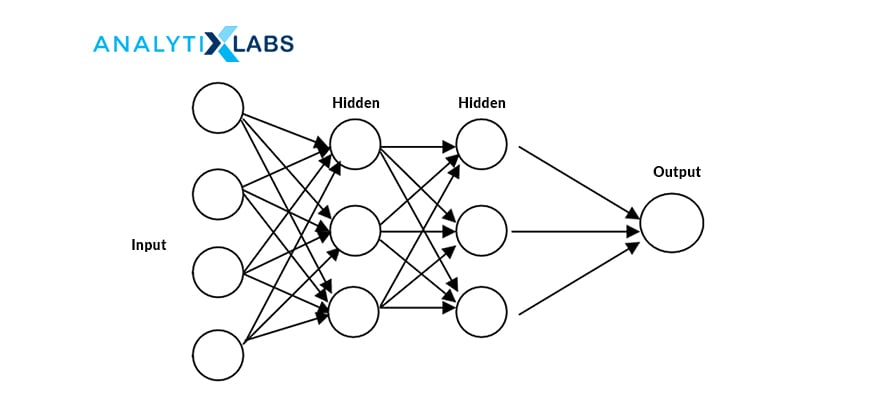
8. What are the components of the neural network?
A neural network has:
- Layers:
- Input Layer: This layer consists of the inputs or the independent X variable.
- Hidden Layer: It is the layer that extracts features and helps with fine-tuning the model
- Output Layer: It is the result or the outcome of the network and depends on the type of business problem.
- Neurons
- Weight and Bias
- Activation Function
Both the hidden and the output layers contain nodes.
9. Explain how a neural network works.
There are three steps to perform in any neural network:
- First, take the input variables and estimate the output or the predicted Y values.
- Next, calculate the loss or the error term, and lastly
- Minimize the loss function or the error term by using the backward propagation, i.e., the loss value is taken backwardly one by one at each layer to update the weights so that the error term is minimized.
10. What role do weights and bias play in a neural network? How are the weights initialized?
Ans. It controls the strength of the connections between the neurons and determines the slope coefficients or the betas. Weights or interconnections transform the input data within the network’s hidden layers. The initial values of the weights (or betas) are randomly assigned between 0 and 1.
Bias is the constant intercept term. It is the model’s default value when no inputs or independent variables are present.
11. What is forward and backward propagation?
Forward and backward propagation is how to adjust the weights or the betas.
In Forward propagation, the weight and biases are randomly initialized. Forward propagation is the process in which the inputs move from the hidden layer to the output layer, i.e., from left to right. The activation function’s output is calculated in every hidden layer until the other layer is processed.
Backward propagation goes in the reverse order. First, adjust the output layer weights (keeping the input and hidden layers weights constant). Relative to the forward propagation, the backward propagation converges faster to the optimal solution of minimized error term since going from the output to the hidden layer and then to the input later.
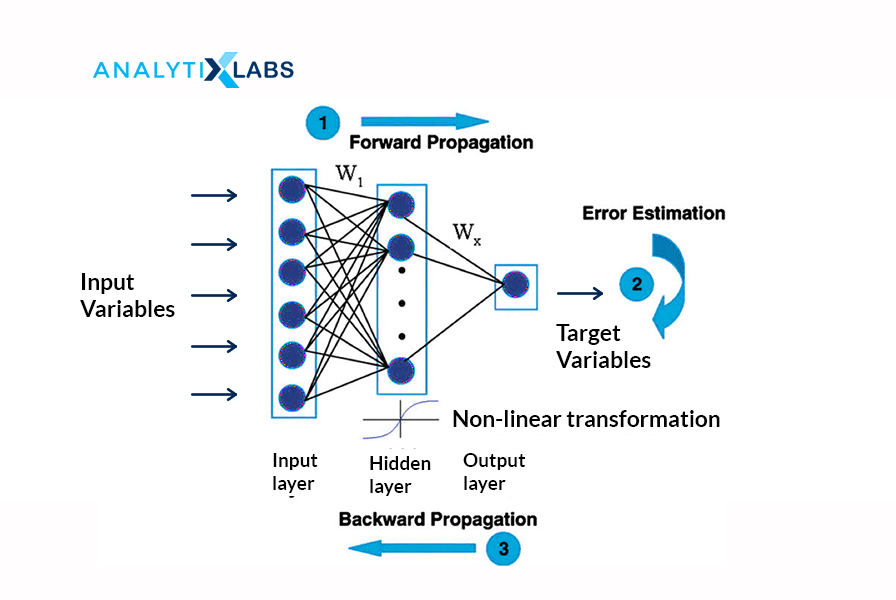
12. What is an activation function, and why is it needed?
Ans. The activation function transforms the inputs into the output by introducing non-linearity to the outcome of a neuron. It helps in deciding to activate or not activate a neuron by calculating the weighted sum with the bias.
Activating a neuron will enable the model output to be nonlinear. It is also known as the squashing function. Some of the commonly used activation functions are:
- Step binary function
- Linear or Identity
- Sigmoid
- Softmax
- Tanh
- ReLU
The objective of the activation function is to add non-linearity to the function.
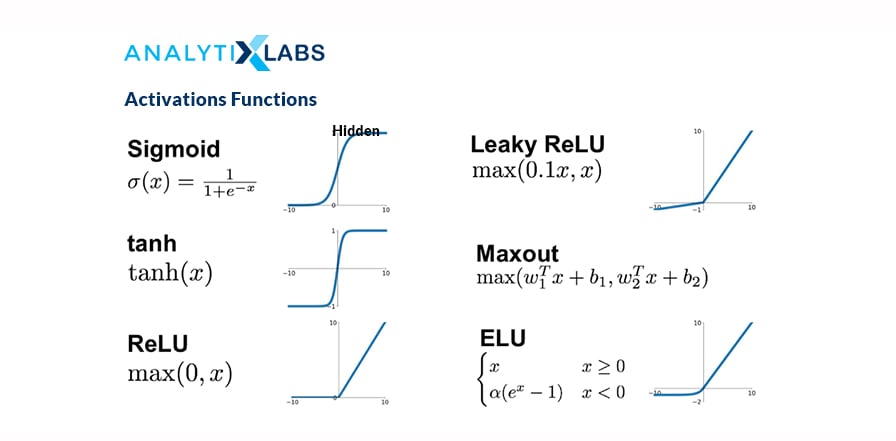
13. Which activation function do we use for each layer?
Ans. The activation function is only applied to hidden and output layers, as the input layer does not have nodes or neurons. We need the outcome in terms of class predicted (1 or 0) on the output layer, so we use sigmoid for binary classification and softmax for multi-classification. We do not need the answer in 1 or 0 for the hidden layer, so use the Rectified linear unit (or ReLU).
14. Why can we not use sigmoid or tanh to activate the hidden layer of the neural network?
The reason for not using Sigmoid or tanh for hidden layers is that these are susceptible to the vanishing gradient problem. By applying to the hidden layers, the gradients get saturated. During the backpropagation process, the error will reduce further (because of the chain rule), and the weights will not get updated, leading to no learning, and eventually, the model will not predict.
15. What is the difference between ReLU and LeakyReLU functions?
ReLU is half rectified from the bottom. In ReLU, the function f(x) = 0 when x< 0 and f(x) = x when x >= 0. This way, in ReLU, the gradient is always zero for all the input values less than zero. It can lead to the ‘dying ReLU’ problem by deactivating the neurons in that region.
On the other hand, the Leaky ReLU function is f(x) = max(0.001x, x) when x < 0, so it will have a slight positive slope (of 0.01) and, therefore, a non-zero value, and consequently, the model continues to learn without hitting a dead-end.
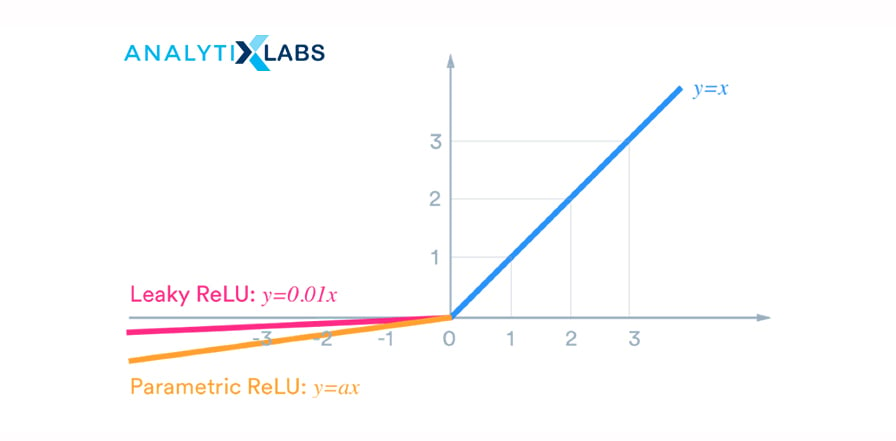
16. What are the challenges with gradient descent, and What are the treatments?
The challenges of Gradient Descent are:
- Gradient descent gets stuck at local minima, and
- It has a constant learning rate
The remedies are:
For the first challenge, use another optimizer called stochastic or mini-batch gradient descent with momentum, and for the second challenge, employ an RMSProp optimizer.
Moving out of the local minima requires accumulating momentum or speed. The gradient descent with momentum calculates the exponentially weighted average sum of the previous gradients and uses this weighted sum to update the weights.
RMSProp scales the learning rate depending on the square of the gradients, which eventually leads to faster convergence to the optimal solution.
Also read: What is Gradient Boosting ALgorithm?
17. What is Adaptive Moment Estimation (or Adam)?
Adaptive Moment Estimation (or Adam) combines the Stochastic Gradient Descent with Momentum and RMSProp. It can adapt the learning rate and find the exponential weights to the historical derivatives. It gives importance to both the past gradients and the previous learning rates.
18. What is the vanishing gradient problem? How is it different from the exploding gradient problem?
Ans. The gradient descent algorithm aims to update the weights and biases of the neural network by taking small steps toward the minimum value of the loss function.
The vanishing gradient problem occurs when these steps towards the optimal solution are too small, thus leading to gradients disappearing. In other words, the changes in weights and bias terms are minimal, almost equivalent to negligible. This way, the model does not learn anything, and the model performance deteriorates.
On the other hand, when the steps are too large, the gradients explode. This enlarges the weights and bias terms updates, leading to an unstable network.
Both of these problems occur when using a Recurrent Neural Network (RNN) since RNNs use backpropagation through time.
19. What are the ways to treat the vanishing and exploding gradient problem?
To fix the vanishing gradient problem, one must use the following:
- ReLU activation function in the hidden layers instead of tanh and sigmoid, and
- Xavier initialization
To treat the exploding gradients problem can:
- Re-design the network with fewer layers.
- Instead of RNN, use the Long Short-Term Memory (LSTM) model
- Use gradient clipping and
- Use the weight regularization
20. What are batch, mini-batch, and stochastic gradient descent? Which one would you use and why?
In a batch gradient descent:
- The gradient is computed for the entire dataset at each iteration. This process is also known as vanilla gradient descent.
- The convergence to the optimal solution is slow as the training data is large, with many samples and many features.
In Stochastic Gradient Descent (SGD):
- The gradient is estimated for a single observation at each iteration.
- Compared to the batch gradient, SGD converges much faster because it updates the weight more frequently and is, therefore, efficient for large data.
Mini-Batch Gradient Descent:
- It is the combination of batch gradient descent and SGD, also known as vanilla mini-batch gradient descent.
- Here, the gradients are estimated by taking one batch or group of observations (similar to SGD for considering one sample) and then, like batch gradient descent, training on this entire batch.
- It works faster than both batch gradient and SGD.
Summarizing the variants of the gradient descents:
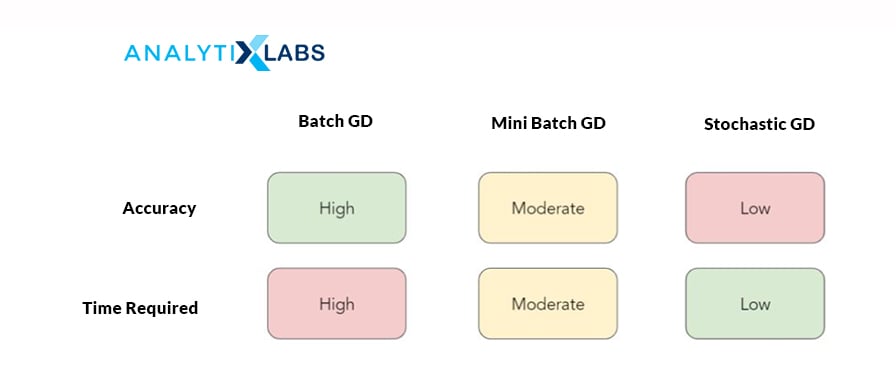
Mini-Batch Gradient Descent is the best variant to use because:
- Using the mini-batches improves the convergence and also avoids the local minima to allow gradient approximation for the entire dataset.
- It is computationally more efficient than stochastic gradient descent.
- It helps improve generalization by finding the flat minima.
21. What is data or image augmentation?
Ans. Data or Image augmentation is a technique for increasing the input data for the model to train and upon. It is done by manipulating the original data. Data augmentation also helps in reducing the overfitting problem.
In image data, an image can be rotated, flipped horizontally or vertically, cropped, converted its channel, added Gaussian blur, scaled, jittering its color, randomly translated, and sheared to create more images used for training.
22. How do you differentiate between CNN and RNN? Which algorithm should be used when?
Convolution Neural Network (CNN) is a type of Feedforward Neural Network (FNN) with convolution as one of its layers. It creates a set of kernels (or filters) that learn and detect features from unstructured data.
CNNs are applicable for performing and analyzing image, signal, and video data. They are used for image recognition and visual imagery analysis.
In a CNN, the signals travel a uni-direction from the input to the output. It takes in only the current input, so it cannot create any network feedback loop and memorize previous inputs.
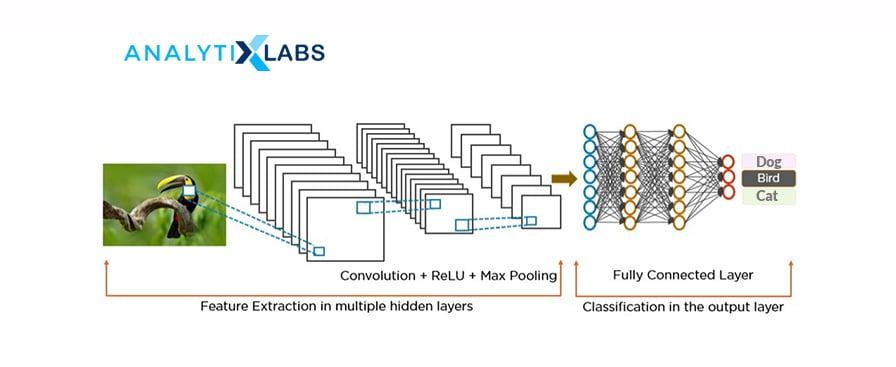
RNNs use Back-propagation Through Time (BTT) to train and store the output of a layer and feed it back to the input layer, enabling it to have an internal state (or memory) for processing the sequences.
Unlike CNN, RNN has interconnected neurons in each layer, and the signals travel in both directions, creating a looped network.
It is used for image captioning, time series forecasting, recognizing handwriting, chatbot creation, fraud, and anomaly detection.
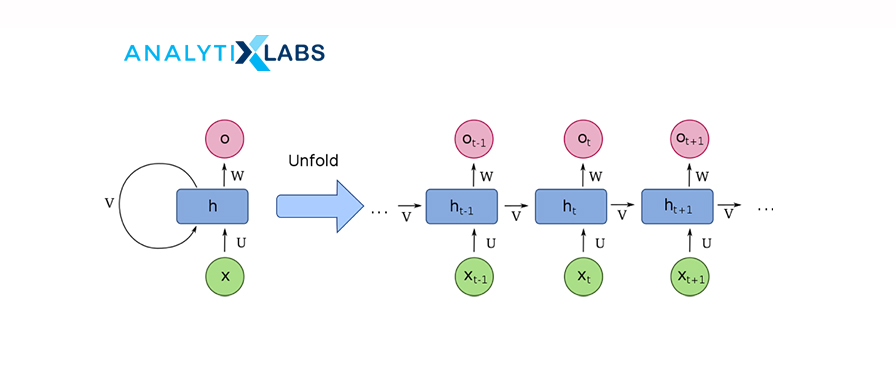
23. Why do we use the Convolutional Neural Network (CNN) for image data and not the Feedforward Neural Network (FNN)?
A convolutional neural network (CNN) is better than a feedforward neural network (FNN) for image data because CNN reads the image in parts rather than taking the entire picture.
CNN has a convolution layer that has filters to build the feature maps. It follows a hierarchical model that creates a network in the shape of a funnel, returns the processed outcome to a densely connected layer in which all the neurons are interconnected, and returns the final image as classified or identified.
This helps share the features parameter, dimensionality reduction, and reduces the computations.
24. Please explain the difference between Conv1D, Conv2D, and Conv3D.
- Conv1D is applicable for input signals similar to the voice i.e audio data.
- Conv2D is useful for images, and
- Conv3D is used for videos having a frame for each period.
25. Explain the different layers of CNN. When using CNN, which layers are the parameters estimated for?
There are four different layers of Convolution Neural Network (CNN):
- Convolutional Layer: The input (images) undergoes a convolution operation. This layer consists of filters (or kernels) that further create a subset of images called feature maps. These maps are used to train the neural network.
- ReLu Layer: It adds non-linearity to the network, converting the negative pixels to zero. It results in a rectified or corrected feature map.
- Pooling: This layer down-samples and reduces the dimensions of the feature maps. It does so by reducing the spatial size of the representation to a lower number of features in patches of the feature map.
- Fully Connected Layer: In this layer, like a feedforward neural network, all the layers are fully connected, meaning the neurons of each layer are connected to neurons of another layer and also have complete activations. This layer identifies and classifies the image.
- Flatten layer: It is connected with the fully connected layer. Flatten converts the data into a one-dimensional array to pass as the input for the next layer.
The parameters are estimated for the convolution and fully connected layer, not the flatter and pooling layer.
26. what is the difference between valid and the same padding in CNN?
Valid padding is used when there is no need for padding. After convolution, the output dimensions of the output matrix are (n – f + 1) X (n – f + 1).
In the Same padding, the elements are added around the edges of the output matrix to keep the dimensions of the input and output matrix the same.
27. What is the difference between dropout and batch normalization?
Ans. Patented by Google, Dropout, and BatchNorm are techniques to improve a deep learning algorithm by reducing the overfitting of the model.
Using the dropout, we can randomly and probabilistically drop or set nodes to zero within layers of a neural network, i.e., effectively making those neurons invisible or “dropped out.” It is a way of creating a different model for each training and averaging the model performance of the network.
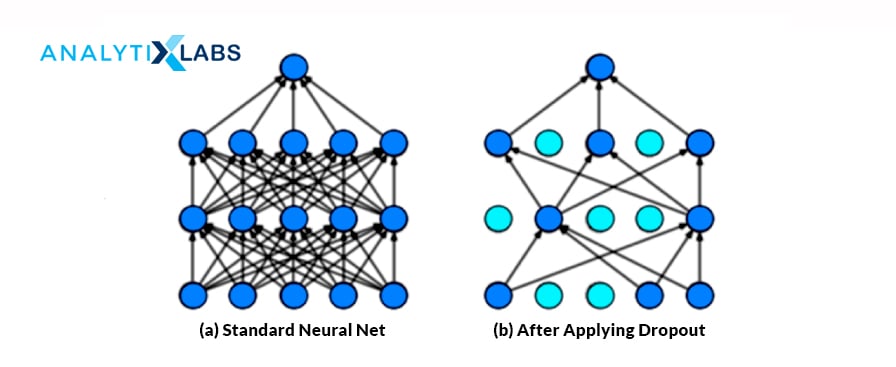
The batch normalization technique standardizes the network’s inputs. It normalizes the hidden layer’s activations with a mean of zero and a standard deviation of each layer. This helps reduce the training time by easily initializing the weights and allowing higher learning rates.
28. What is transfer learning? Why is it needed? Name commonly used transfer learning models.
Transfer learning is the process of learning from a model to be transferred to another without needing to train the model from scratch. There are three ways to use transfer learning:
- Extract features from the pre-trained model and also remove the output from the pre-trained model, using it on the data for the current problem.
- Use both the architecture and weights of the pre-trained model and take that to train on our dataset.
- Train some layers and freeze the other layers of the pre-trained model. This works by extracting the weights of some of the layers and uses for our neural network and fine-tuning the model.
The most commonly used transfer learning models are:
- ResNet
- VGG-16
- GTP-2 and GPT-3
- BERT
29. Does the problem of overfitting happen in neural networks? If yes, then how would you treat it?
Ans. Yes, overfitting can occur in a neural network. Following are the ways to prevent overfitting in a neural network or improve a deep learning algorithm:
- Early Stopping
- Dropout
- Batch Normalization
- Data or Image Augmentation
- Weight Sharing
30. Do we have regularizers for neural networks?
Yes, the dropout is a regularizer.
31. What are hyperparameters? List the hyperparameters used in a neural network.
It helps to determine the structure of the neural network and how the network will be trained. Hyperparameters are the variables that are set before the training starts. These values cannot be learned from the data and must be defined by the user. The hyperparameters of an artificial neural network are:
- Number of hidden layers
- Number of Nodes in the hidden layer
- Number of epochs (or iterations)
- Batch size
- Optimizer
- Activation Function
- Learning Rate
- Momentum
- Network Weight Initialization
32. Explain how LSTM and GRU work. Which is the best one to use and why?
Long-short-term memory (LSTM) is a variant of the recurrent neural network that can learn long-term dependencies. It uses three gates: a forget gate, an input and output gate, and standard units to include a ‘memory cell’ that helps maintain the information in memory for long periods. It uses the feedback loop and gates to “remember” and “forget” information.
The LSTM network works in the following manner:
- Step 1: The network picks and decides which information to remember and what to forget.
- Step 2: It selectively updates the cell state values based on the first step.
- Step 3: The network calculates to decide which part of the current state should be output.
Gated Recurrent Unit (GRU) is a special case of LSTM. It uses two gates: a reset gate and an update gate. The reset gate decides how to combine the new input with the previous time steps’ memory. The update gate decides how much of the last memory should be kept. The update gate of GRU combines the input and forget gate of LSTM.
GRU is preferred over LSTM as LSTM can become complex by having an additional gate. GRU also resolves the vanishing gradient problem, operates faster, and consumes less memory as it does not have internal memory.
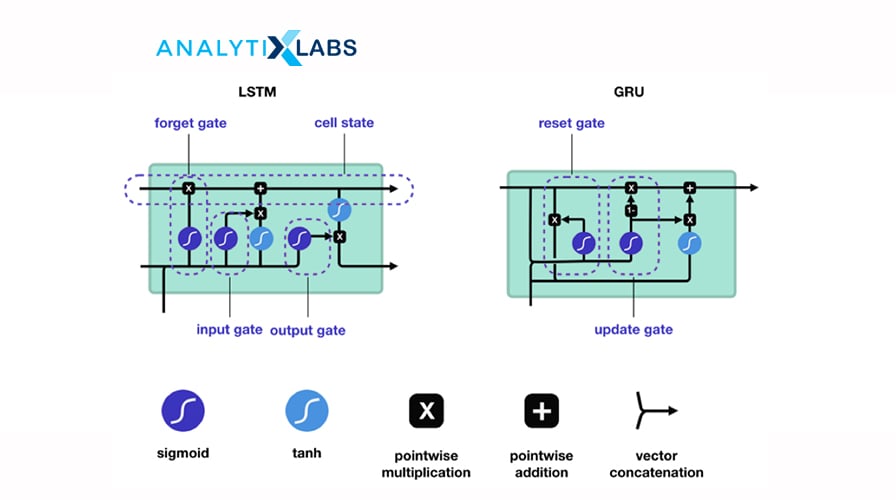
33. What are Autoencoders? What is the use of Autoencoders?
Autoencoders is an unsupervised deep learning algorithm. Its goal is to automatically learn to map the inputs to the corresponding outputs without any supervision or direction. It comprises two parts (which are different from the Encoder-Decoder model):
- Encoder: fits the input into an internal representation
- Decoder: converts the internal computational states back into the output
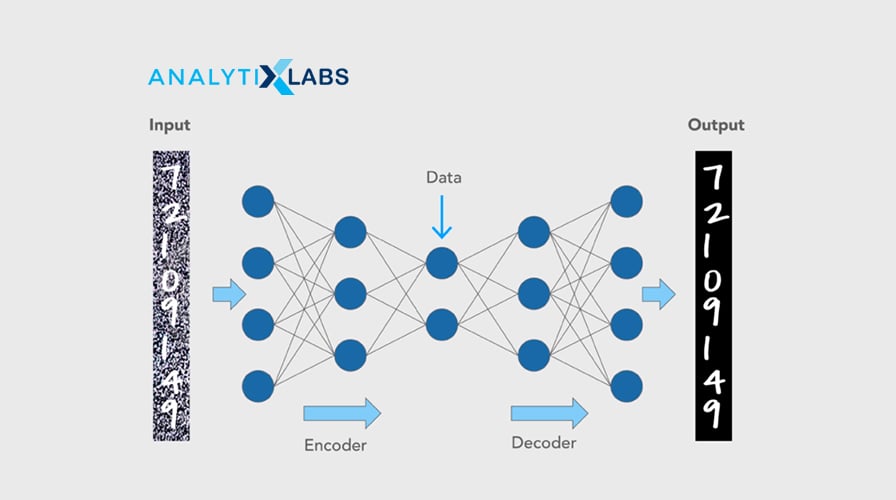
Autoencoders are primarily used for dimensionality reduction, decreasing the size of the inputs into a smaller representation. They are also used for image reconstruction, denoising images, and image colorization.
34. What are GANs?
Generative adversarial networks (GANs) are unsupervised algorithms that use two neural networks: one generator and a discriminator. It generates data with the intent to uncover patterns to create the output.
The generator generates new data, and the discriminator classifies the generated data. Both parts are trained simultaneously and compete with each other.
GANs are popular with image data as they are highly efficient and have high traction. These are used in image generation, image enhancement, translation, video, voice generation, and age progression.
35. Explain the Boltzmann machine. What is a Restricted Boltzmann machine?
Ans. The Boltzmann Machine resembles a Multilayer Perceptron with a hidden and visible input layer. This model makes binary decisions and bias, i.e., it takes stochastic decisions to decide if a neuron must remain on or off. These generative models are bidirectional and stochastic models where nodes across different layers can connect; however, two nodes within the same layer can not connect.
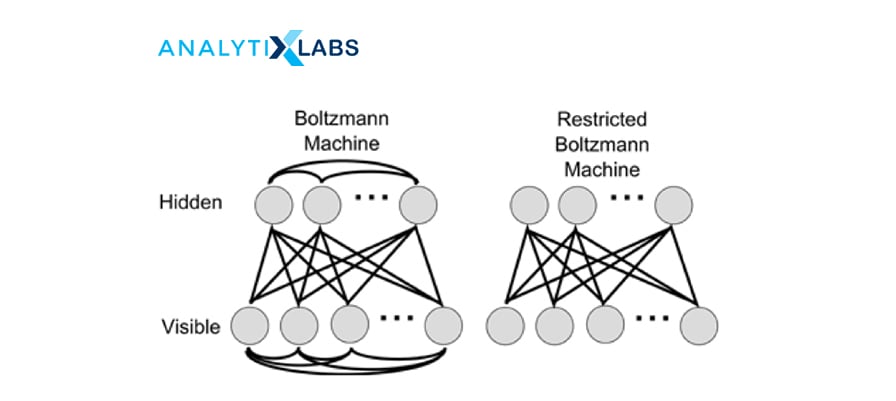
A Restricted Boltzmann Machine (RBM), a variant of the Boltzmann machine. It is an undirected graphical model that can be trained in supervised or unsupervised ways based on the task. It is an algorithm that is used to perform:
- Regression
- Collaborative filtering
- Classification
- Dimensionality reduction
- Feature Learning
- Topic modeling
A Restricted Boltzmann Machine is a Boltzmann Machine with restrictions. The restrictions are the visible nodes that should not connect with each other and the hidden nodes that shouldn’t connect with each other.
36. What are Seq2Seq (encoder-decoder) models? How is it different from Autoencoders?
The encoder-decoder model is a recurrent neural network (RNN) architecture with two RNNS and is used for sequence problems such as machine translation. One part is the encoder, which encodes the inputs into a fixed-length vector representation. The second part is the decoder, which decodes or represents another series of symbols as the output.
It differs from the autoencoder as it is an unsupervised architecture focussing on reducing dimensions and is applicable for image data.
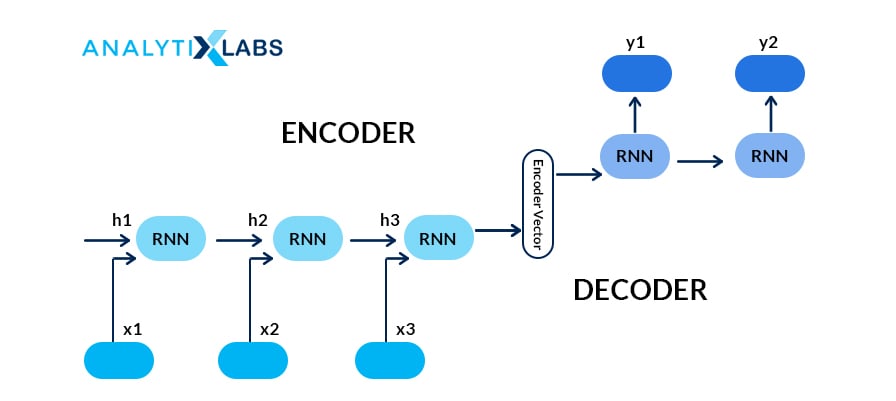
Source: miro.medium.com
37. What is the difference between epoch, batch, and iteration in deep learning? If we have a dataset with 10,000 records and a batch size of 100, then how many iterations will our model run for?
Epoch is the number of iterations over which we train the model. Batch refers to the size of the subsets that the dataset is divided into to pass in the model.
The model will run 100 iterations ( (10,000 divided by 100) for 1000 records and have a batch of 100.
38. What are tensors?
Tensors are mathematical objects, and in deep learning, they are one of the data structures. These multidimensional arrays represent data with higher dimensions, and one can execute various mathematical operations on them. In other words, tensors are data containers that store data with different dimensions in a neural network.
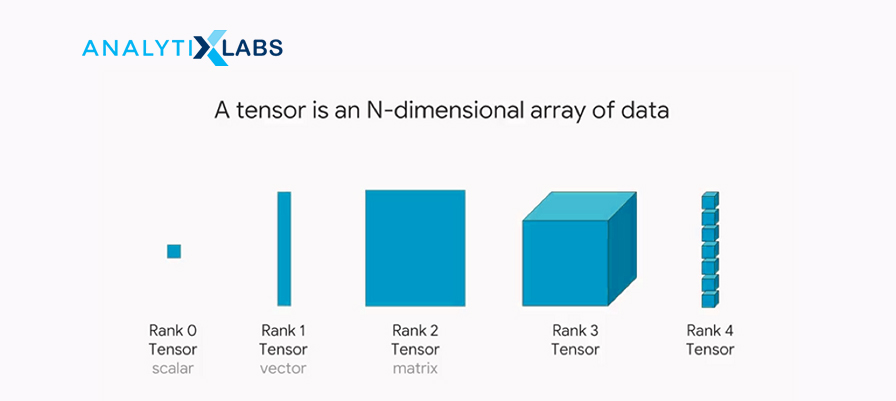
Source: miro.medium.com
To explore tensors more, read Tensors — Representation of Data In Neural Networks and TensorFlow Basics: Tensor, Shape, Type, Sessions & Operators.
39. What is a computational graph? How is it useful for Deep Learning?
A computational graph is a series of operations represented in a network form, where each node represents a mathematical operation and the edges are the tensors. Each node takes input as one or more tensors and returns a tensor as output. This sequence of TensorFlow operations is also known as the ‘DataFlow Graph.’
The USP of this graph is that processing can happen parallelly, making it computationally efficient. The graph is immensely helpful for deep learning, as it takes in a large number of inputs and has multiple layers requiring more computations. It can chart out the mathematical workflow graphically.
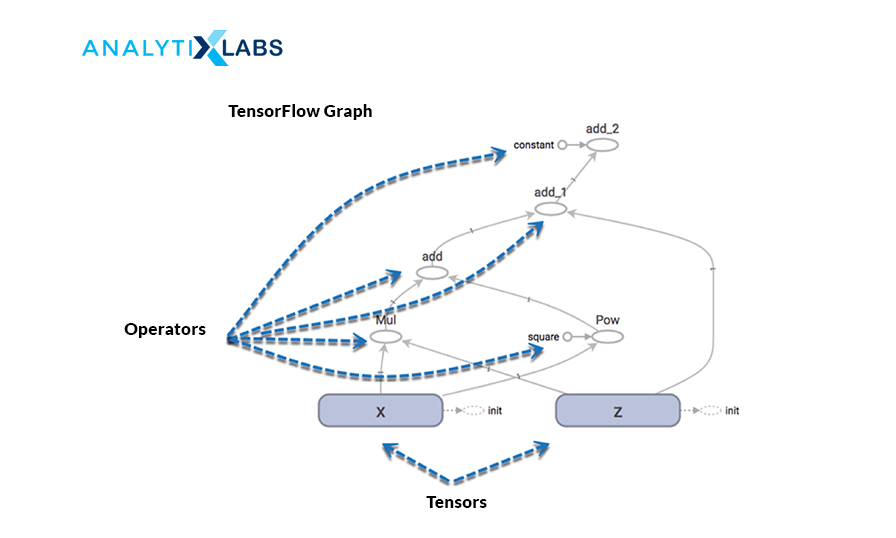
Ans. Model capacity refers to the degree of a deep learning neural network that controls the types of mapping functions it can take and learn from. It is the ability to approximate any given function. The higher the model capacity, the more information can be stored in the network.
41. Explain the Learning Rate in a neural network model. What are the consequences of it being too low or high?
The stochastic gradient descent algorithm is used to train deep-learning neural networks. The algorithm uses examples from training data to approximate the error gradient for the present state. It then updates the model weights using back-propagation to reduce the error estimates in the loss function. The total number of weights updated in this training is termed learning rate or step size.
You can mention the following points for learning rate:
- It is a configurable hyper-parameter used in training neural networks with small positive values ranging between 0 and 1.
- It determines how the model can be adapted to the given test problem. So, testing and tuning the selected value of the learning rate is necessary.
- In a particular training cycle, a perfectly attuned learning rate is suitable to optimally estimate the function with the provided resources (no. of layers and nodes in each layer).
- If the learning rate is too low, the neural network optimizer will take longer to reach the point of minimized loss and will converge on an undesirable sub-optimal value.
- On the contrary, choosing a high learning rate value can lead to overshooting, as the algorithm can hop to the minima quickly, causing large weight updates.
42. What is Convux Hull? Where is it used?
The Convex Hull encloses all the convex sets in an Euclidean space. The smallest polygon covers all the points for a given set. In data science, convex hulls are used in image processing, image recognition, classification, and collision avoidance.
43. What would be the consequences of removing activation functions from a neural network?
Activation functions add non-linearity to neural networks, enabling them to learn complex operations. Removing activation functions would convert the network into a simple linear regression model, as neurons will perform only linear transformations based on biases and weights.
44. Can a neural network be trained by initializing all the weights to 0 or a constant?
The Zero or constant initialization method allows the neurons to learn similar features and produce the same calculations in each training iteration. Thus, the derived gradients will remain the same in all the propagations, and neurons will learn identical things.
45. What is Bottleneck in Autoencoders? Why is it used?
The bottleneck is a lower-dimensional hidden layer used to reduce the input size to make it easier for the model parameters to learn. It allows compressed knowledge representation of the original input, i.e., filtering relevant information features and discarding irrelevant information.
46. How can a Tanh activation function help the neural network converge faster than a logistic sigmoid?
Both Tanh and sigmoid functions are S-like, which means their input values are suppressed to a defined range. However, the behavior of the activation functions is varied by their gradient in the region near zero.
When using the Tanh function, the gradient is quadruple times greater than that of the sigmoid, and the difference of the output is symmetric around zero, having values -1 and +1. This results in faster convergence to the optimal minima. Thus, Tanh outperforms sigmoid activation when we need big learning steps.
47. Define Fine-tuning. Why is it used in Deep Learning?
Fine-tuning is a technique for model reuse that utilizes transfer learning. The technique uses unfreezing some layers on the topmost layer of the model library. In other words, the data from the existing neural network is used as an initial point for training new neural network models. This is done to undergo feature extraction and train the upcoming sets of the model.
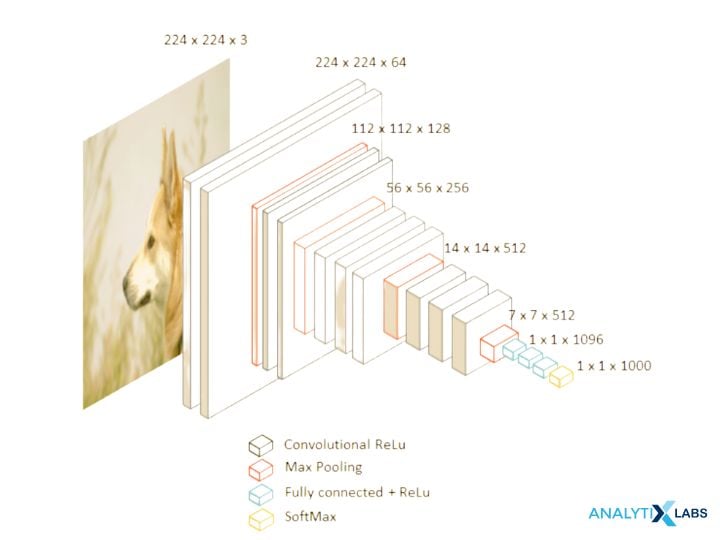
Advantages of Fine Tuning:
- Fine-tuning utilizes a trained network model to perform a similar task.
- Eliminates the hassle of building the feature extraction network from the initial level.
- Provides the optimal benefit of the feature extraction done previously in models.
- Resource efficiency and reduces the model training time.
Fine-tuning steps are:
- Import the data from the pre-trained system.
- Replace the previous system’s output layer, as it is unusable for the new model.
- Modify and freeze the layers according to the requirements of the new model.
- Modify the input layer to train the new neural network model.
48. What is pooling? How does it help in filtering features?
Convolution Neural Network architectures include a feature called pooling that aims at accumulating attributes from the maps produced by the convolution of a filter on an image. Its purpose is to shrink the spatial volume of the image representation progressively. It decreases the computational cost in the neural network and reduces the number of parameters. Max pooling is the one in which the largest pixel values are selected from the segment. The other two types are mean and average pooling.
49. What are ensemble learning methods?
A machine learning technique that combines different base models to generate an optimal predictive model. It merges the predictions from several NN models to minimize the inconsistency of predictions and decrease the generalization error.
Random forest models and BAGGing models are the types of ensemble methods.
50. How can a Dense Layer of a CNN convert into a Fully Convolutional Layer?
It is one of the popular CNN interview questions.The conversion steps are:
- Replace the lowest dense layer with a convolutional layer with a kernel size equal to the input size of the layer. Use a single filter per neuron in the dense layer and apply valid padding.
- Set the activation function the same as the dense layer’s.
- Convert other dense layers by using 1×1 filters.
- Reshape the weights of the dense layers to convert a trained CNN to FCN.
51. What is the most interesting project you have worked on?
Provide a technical description of the project and your contribution to it. Try to highlight your skills and strengths while including adjectives describing your character.
52. How would you use backpropagation?
Setting the Model components for Backpropagation requires
- Training deep neural network model using the exclusive OR (XOR) function
- Determining the activation value of each node using the activation function
- Determining the input to the activation function using the hypothesis function
- Estimating the cost function of logistic regression using the loss function
- Determining the direction to adjust the weights using the batch gradient descent optimization function.
Beginner-level Deep Learning Interview Tips
It would be beneficial to do the following to prepare for the deep learning interview:
- Having good theoretical knowledge
It is imperative to have sound, solid theoretical knowledge of deep learning topics. Starting from the basics of neural networks, their architecture, and the several parameters used in them, a good working understanding of the commonly used models such as Convolution Neural Network (CNN), Recurrent Neural Network (RNN), Long-Short-Term Memory (LSTM), and Gated Recurrent Unit (GRU).
Be thorough with how the models help solve a business problem, how to improve the neural network, and the respective hyperparameters. Additionally, you need to know what transfer learning is, why it is needed, and how it is employed. Be well-versed with the fundamentals of the underlying concepts used in the models. Keep revisiting these deep learning interview questions to revise your concepts.
- Work with real-world Deep Learning cases
Nothing beats the old-age saying practice makes a man perfect! All the theories are not of any good if they are not applied. It is crucial to have worked with business cases to solve any real-life problems. It is best to showcase your work and skills with a portfolio on GitHub and/or Kaggle.
- Study Deep Learning interview questions
The above deep learning interview questions and answers would be a good starting point to study. Practice your answers; take mocks. It would save you many rounds of drilling work if you could hear yourself when you answer a question! Technical rounds can get a little daunting at times. In such cases, keep a mind hack trick handy to swift through it.
- Read about the Company and Role
It is an excellent practice to research and read well beforehand the job description, about the company, and if possible, know its contemporaries. Be aware of the needed skill sets and tools, talk about how you have those skills, and work on cases requiring those tools (only if you have them!) Assess and answer your questions, such as telling us about yourself and what you can bring to the role. Have a list of questions to ask the interviewer at the end of the interview.
- Interview Étiquettes
Some of the very subtle and essential things that we can tend to forgo while preparing for interviews are:
- Being punctual for the interviews, both virtual and in-person. Logging in at least a couple of minutes before time will allow you to breathe in, be settled, collect your thoughts, and not rush hush through (after all, a good start is half done 🙂
- Being honest and integral about what you know and don’t know. On your resume, add only the things you know and have worked on yourself.
- Listen to the interviewer and answer questions to the best of your ability and understanding. Ask if you are not clear about the question. Answer only to the question asked. Sometimes, we tend to answer for what was not even asked. If you are unsure or do not know the answer, it is polite to say I don’t know rather than conjuring up incorrect answers.
How To Become an Expert in Deep Learning?
You can start with our Deep Learning Certificate Course. Our certifications course has no prerequisites, making it easy for you to start learning. If you have experience in this domain, the initial sessions will work as a revision for you. The course curriculum follows a progressive learning approach, helping learners adapt to the pace and learn the modules sincerely.
Explore our Deep Learning With Python Course today. Club your learning with additional courses on AI Engineering and ML with Python.
- Having a Beginner’s Mind
We all want to leverage the benefits of this highly sort demanding and lucrative job and build fantastic working models, but Rome was not built in a day. It takes work to climb up the ladder and break through this field like any other.
First, work with the basics, get your fundamentals in place, and then progress to the advanced topics in deep learning, such as transformers, machine translations, speech-to-text, applied computer vision, BERT, and YOLO.
- Do not forget your Machine Learning Stuff!
If you were expecting to let your Machine Learning books, notes, and models collect dust, I’m sorry to disappoint you. You can’t proceed with deep Learning until you have Machine Learning under your belt!
Deep Learning without Machine learning would be like you have become a doctor without having had science in 12th class! (No, no, Munna-Bhai MBBS works here!) There is a reason why Deep Learning is a subset of Machine Learning, and it has to be the last one to be learned and mastered. The pecking order of things is important.
- Pick your Deep Learning framework
There are three popular frameworks for working with deep learning problems: Keras, TensorFlow, and PyTorch. Keras makes it easier to start working on and implementing the Deep Learning models as it has pre-defined functions, as sklearn had for Machine Learning. Keras has TensorFlow at its backend.
PyTorch requires more work, as we need to define functions for the same task that is handier in Keras. Ideally, start with Keras, and once you have a grasp of the models, clear on the architecture et al., learn PyTorch and diversify out.
- Practice, Practice, and some more Practice!
It would be good to have intuition built into neural networks, a logical understanding of things, and how the pieces are joined together. The key is the curiosity to ask questions, understand, learn, and then apply all that knowledge gained. It is one thing to understand the architecture of the network, but only once you start putting that into solving real-life cases will you be able to learn more and fine-tune your approach.
- Explore the problems and datasets
Play around with every kind of dataset you can get hands-on, and over time; you will learn which area of Deep Learning you have flair and liking for. This can only come up with having worked with many problems and diversifying the use cases. It is exactly like how you get to know yourself 🙂
- Learn Model Deployment
Eventually, the models you are making must be deployed for them to be useful. Therefore, learning how to deploy your models is immensely beneficial. The skill will give you an added advantage and make you more efficient.
Hope this repository of deep learning interview questions has been helpful to you. Some of the FAQs related to it are:
For hands-on AI projects, also refer to 18 (Interesting) Artificial Intelligence Projects Ideas
FAQs – Frequently Asked Questions
What are the topics in Deep Learning?
The topics in Deep Learning are:
- Basics of Neural Networks
- Perceptrons
- Multi-Layer Perceptron
- Forward and Backward Propagation
- Gradient Descent Algorithm
- Loss Function
- Activation Functions
- Optimizers
- Convolutional Neural Networks (CNN)
- Recurrent Neural Networks (RNN)
- Long-Short Term Memory (LSTM)
- Gated Recurrent Unit (GRU)
- Autoencoders
- Encoder-Decoder
- Attention Models
- Transformers
- Restricted Boltzmann Machine (RBM)
- Speech-to-Text Analytics
- Image, Text, and Audio Processing
- Computer Vision
- Transfer Learning
- Techniques to improve the Deep Learning model
- Deep Learning Frameworks such as Keras, TensorFlow, PyTorch
How do you prepare for a deep learning interview?
To prepare for deep learning interview questions, you must have a very sound theoretical knowledge of the basics of neural networks, the terminologies involved in them, and the different algorithms used covered in the topics of deep learning above. Go over the deep learning interview questions.
You must also have an end-to-end working knowledge of the algorithms themselves. Be thorough with a deep learning project’s workflow and be ready to walk through your best or favorite deep learning use case during the interview. Having a repository to showcase your work and a portfolio on Github, Kaggle, or both is an added advantage.
You may also like to read:
1. Top 50 Data Science Interview Questions And Answers
2. Top 60 Artificial Intelligence Interview Questions & Answers
3. Top 75 Natural Language Processing (NLP) Interview Questions